对于线性关系,我们可以进行简单的线性回归。对于其他关系,我们可以尝试拟合一条曲线。
曲线拟合是构建一条曲线或数学函数的过程,它对一系列数据点具有最佳的拟合效果。
使用示例数据集
#我们将使Y成为因变量,X成为预测变量
#因变量通常在Y轴上
plot(x,y,pch=19)
可下载资源
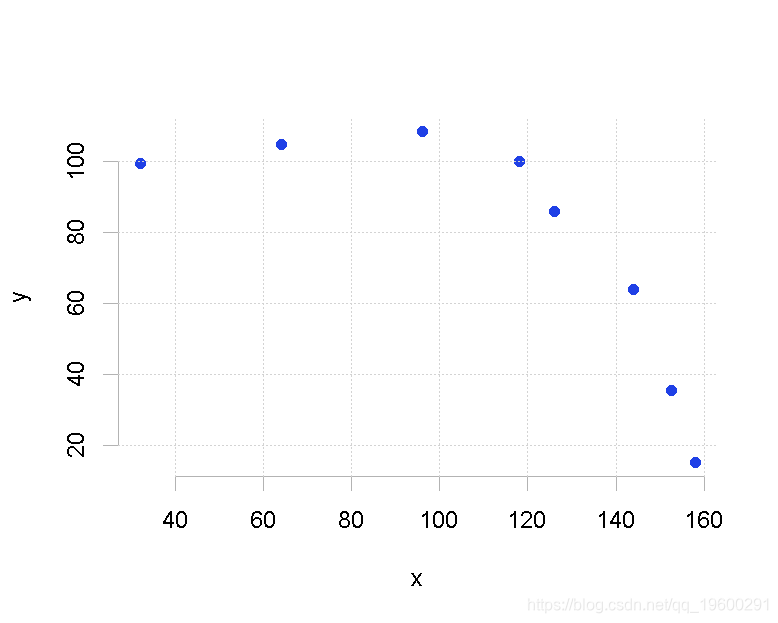
看起来我们可以拟合一条曲线。
#拟合一次多项式方程。
fit <- lm(y~x)
#二次
fit2 <- lm(y~poly(x,2)
#三次
......
#生成50个数字的范围,从30开始到160结束
xx <- seq(30,160, length=50)
lines(xx, predict(fit, xx)
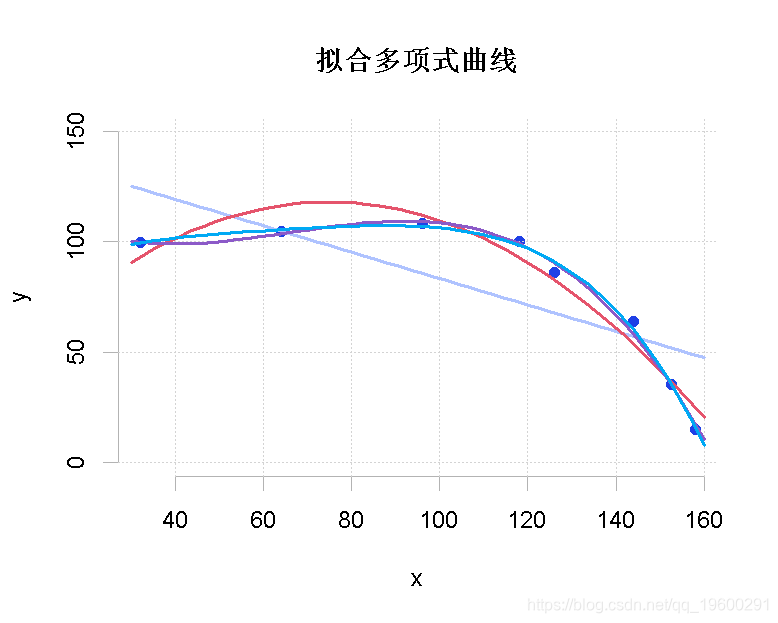
我们可以看到每条曲线的拟合程度。
我们可以使用summary()函数对拟合结果进行更详细的统计。
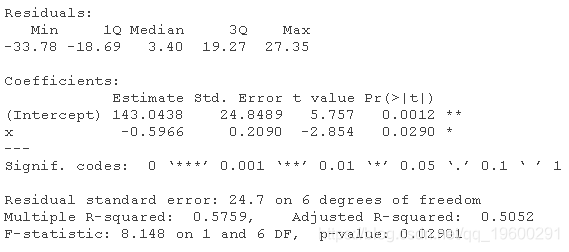
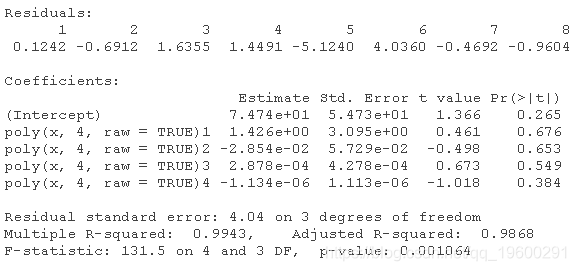
使用不同多项式R平方的总结。
1st: 0.5759
2nd: 0.9474
3rd: 0.9924
4th: 0.9943
我们可以用 “方差分析 “来比较不同的模型。
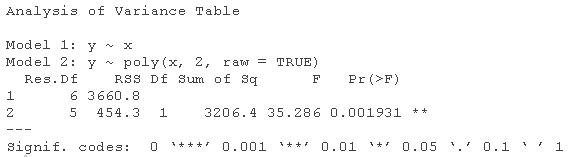
Pr(>F)值是拒绝无效假设的概率,即一个模型不比另一个模型更适合。我们有非常显著的P值,所以我们可以拒绝无效假设,即fit2比fit提供了更好的拟合。
随时关注您喜欢的主题
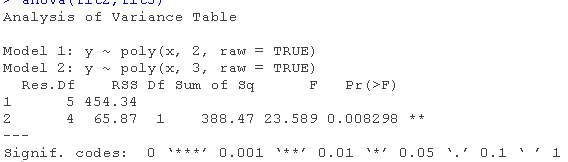
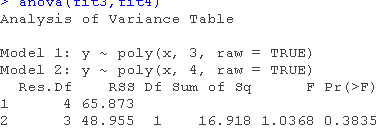
我们还可以创建一个反映多项式方程的函数。
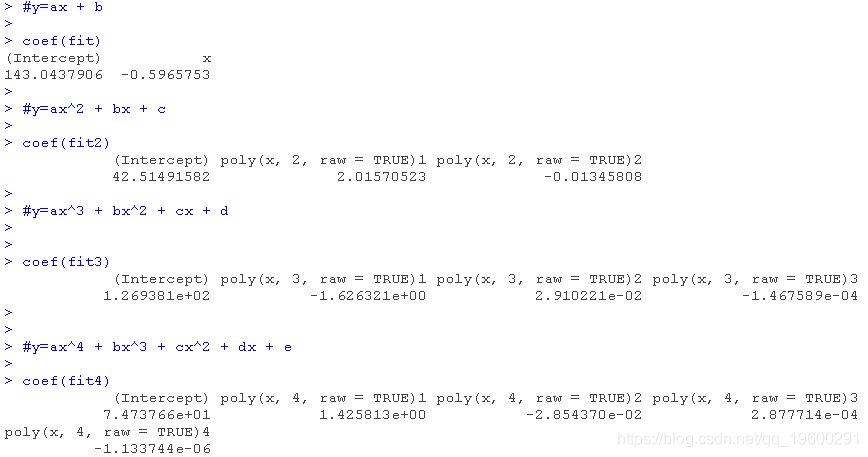
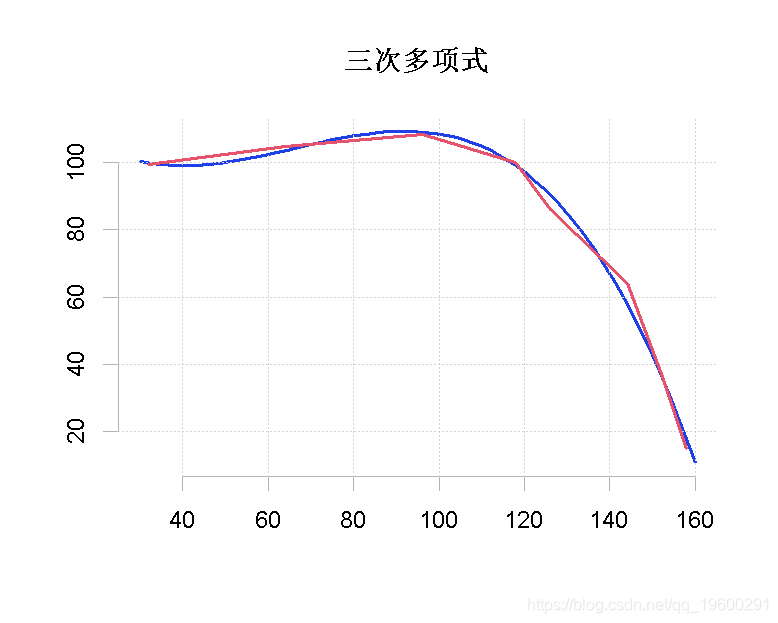
从三次多项式推算出来的数值与原始数值有很好的拟合,我们可以从R-squared值中得知。
结论
对于非线性曲线拟合,我们可以使用lm()和poly()函数,这也为多项式函数对数据集的拟合程度提供了有用的统计数据。我们还可以使用方差分析测试来评估不同模型之间的对比程度。从模型中可以定义一个反映多项式函数的函数,它可以用来推算因变量。
yy<-third(xx,fit)
plot(xx,yy)
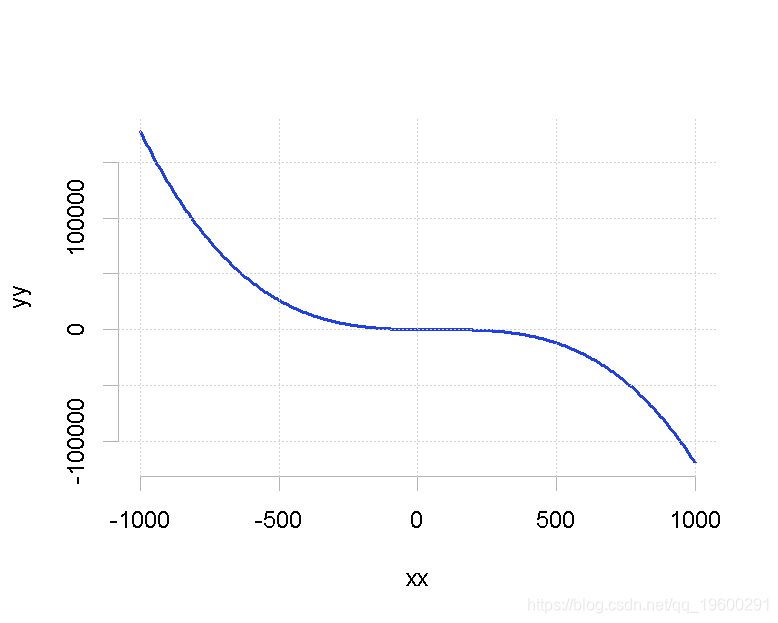