然而,乍一看,y的水平在中间移动,所以它似乎并不总是有稳定的关系(背后有多个状态)。
可下载资源
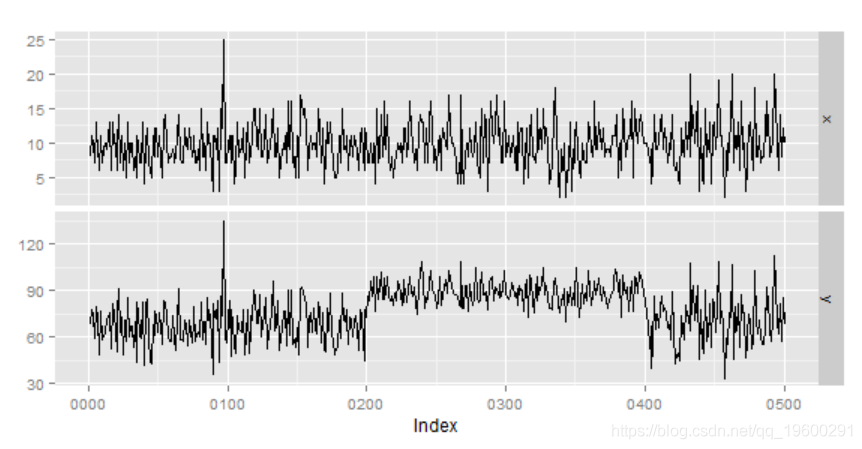
上面的样本数据创建如下。数据根据时间改变x和y之间的关系。
x <- rpois(500, lambda = 10)
y1 <- x * 4 + 20
y2 <- x * 2 + 60
noise <- rnorm(1:500, mean = 10, sd = 5)
y1 <- y1 + noise
y2 <- y2 + noise
y <- c(y1[1:200], y2[201:400], y1[401:500])
observed <- data.frame(x = x, y = y)
x和y1,y2之间的关系如下图所示。如果您知道x和y有两种状态,则x和y看起来像这样。
数据
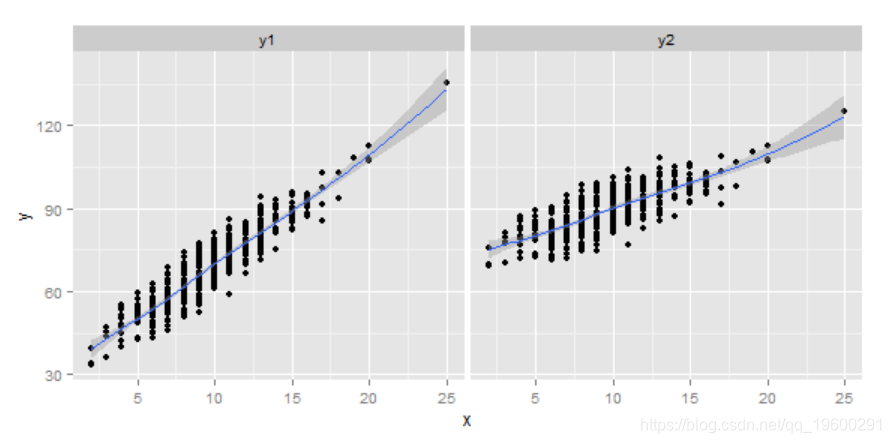
在马尔可夫转换模型中,观察数据被认为是从几个状态生成的,并且如上所示很好地分离。
观察到的数据
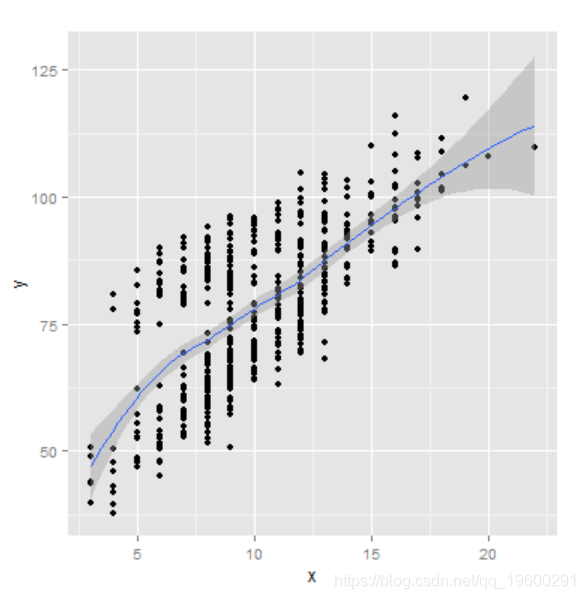
创建马尔可夫转换模型
模型公式
# Call:
# lm(formula = y ~ x, data = observed)
#
# Residuals:
# Min 1Q Median 3Q Max
# -24.303 -9.354 -1.914 9.617 29.224
#
# Coefficients:
# Estimate Std. Error t value Pr(>|t|)
# (Intercept) 45.7468 1.7202 26.59 <2e-16 ***
# x 3.2262 0.1636 19.71 <2e-16 ***
# ---
# Signif. codes:
# 0 ‘***’ 0.001 ‘**’ 0.01 ‘*’ 0.05 ‘.’ 0.1 ‘ ’ 1
#
# Residual standard error: 11.51 on 498 degrees of freedom
# Multiple R-squared: 0.4383, Adjusted R-squared: 0.4372
# F-statistic: 388.7 on 1 and 498 DF, p-value: < 2.2e-16
参数的含义是
# Markov Switching Model
#
# AIC BIC logLik
# 3038.846 3101.397 -1513.423
#
# Coefficients:
#
# Regime 1
# ---------
# Estimate Std. Error t value Pr(>|t|)
# (Intercept)(S) 69.3263 4.0606 17.0729 <2e-16 ***
# x(S) 2.1795 0.1187 18.3614 <2e-16 ***
# y_1(S) -0.0103 0.0429 -0.2401 0.8103
# ---
# Signif. codes: 0 ‘***’ 0.001 ‘**’ 0.01 ‘*’ 0.05 ‘.’ 0.1 ‘ ’ 1
#
# Residual standard error: 4.99756
# Multiple R-squared: 0.6288
#
# Standardized Residuals:
# Min Q1 Med Q3 Max
# -1.431396e+01 -2.056292e-02 -1.536781e-03 -1.098923e-05 1.584478e+01
#
# Regime 2
# ---------
# Estimate Std. Error t value Pr(>|t|)
# (Intercept)(S) 30.2820 1.7687 17.1210 <2e-16 ***
# x(S) 3.9964 0.0913 43.7722 <2e-16 ***
# y_1(S) -0.0045 0.0203 -0.2217 0.8245
# ---
# Signif. codes: 0 ‘***’ 0.001 ‘**’ 0.01 ‘*’ 0.05 ‘.’ 0.1 ‘ ’ 1
#
# Residual standard error: 4.836684
# Multiple R-squared: 0.8663
#
# Standardized Residuals:
# Min Q1 Med Q3 Max
# -13.202056966 -0.771854514 0.002211602 1.162769110 12.417873232
#
# Transition probabilities:
# Regime 1 Regime 2
# Regime 1 0.994973376 0.003347279
# Regime 2 0.005026624 0.996652721
输出中的制度1和制度2表示后面的两个状态 。
# Regime 1
# ---------
# Estimate Std. Error t value Pr(>|t|)
# (Intercept)(S) 69.3263 4.0606 17.0729 <2e-16 ***
# x(S) 2.1795 0.1187 18.3614 <2e-16 ***
# y_1(S) -0.0103 0.0429 -0.2401 0.8103
y1 <- x * 4 + 20
可以看到Regime 2 与之兼容。
随时关注您喜欢的主题
可以说从调整后的R平方值整体上有所改善。
# Regime 2
# ---------
# Estimate Std. Error t value Pr(>|t|)
# (Intercept)(S) 30.2820 1.7687 17.1210 <2e-16 ***
# x(S) 3.9964 0.0913 43.7722 <2e-16 ***
# y_1(S) -0.0045 0.0203 -0.2217 0.8245
模型
对于每个regime,目标变量+指定的解释变量和处于该状态的概率以阴影绘制
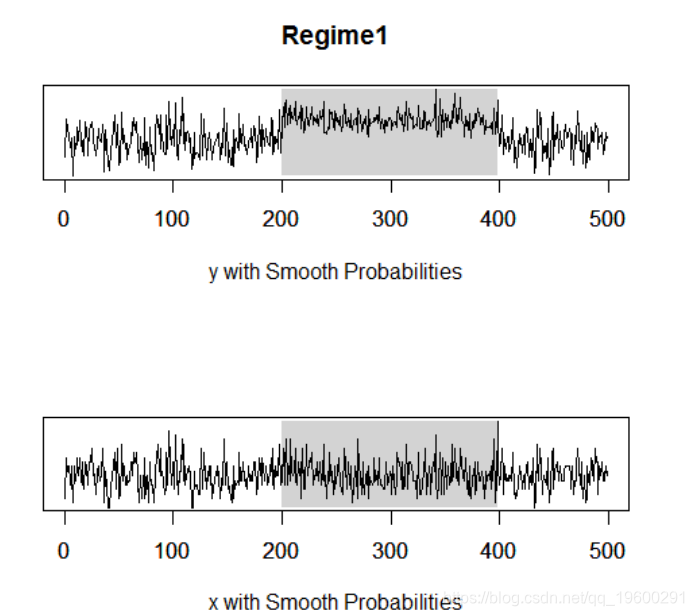
每个时间点的概率
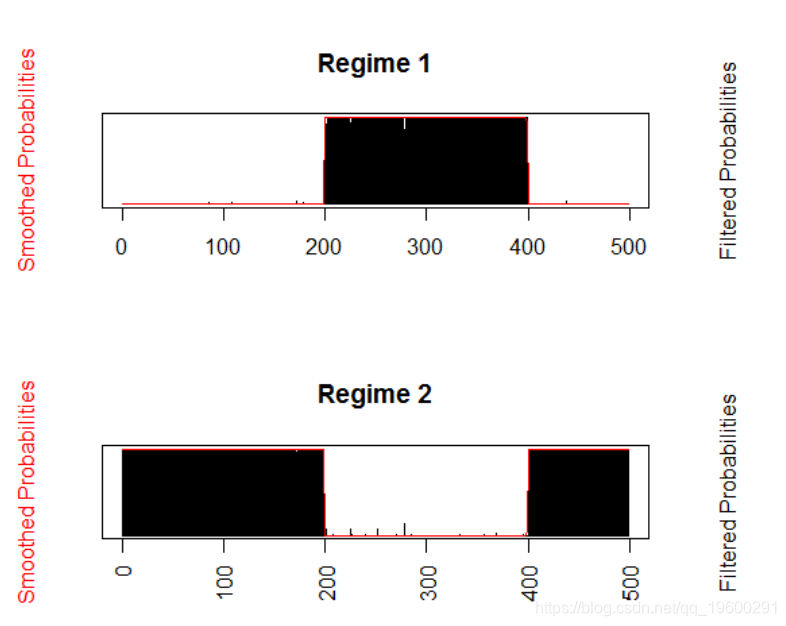
每次获取状态和更改点
如果你想知道你在某个特定时间点所在的regime,那么就选择那个时刻概率最高的 。
> probable
[1] 2 2 2 2 2 2 2 2 2 2 2 2 2 2 2 2 2 2 2 2 2 2 2 2 2 2 2 2 2
[30] 2 2 2 2 2 2 2 2 2 2 2 2 2 2 2 2 2 2 2 2 2 2 2 2 2 2 2 2 2
...
异常值/变化点是Regime更改的时间
c(FALSE, diff(probable) != 0)
[1] FALSE FALSE FALSE FALSE FALSE FALSE FALSE FALSE FALSE FALSE
[11] FALSE FALSE FALSE FALSE FALSE FALSE FALSE FALSE FALSE FALSE
...
[181] FALSE FALSE FALSE FALSE FALSE FALSE FALSE FALSE FALSE FALSE
[191] FALSE FALSE FALSE FALSE FALSE FALSE FALSE FALSE FALSE TRUE
[201] FALSE FALSE FALSE FALSE FALSE FALSE FALSE FALSE FALSE FALSE
...
[381] FALSE FALSE FALSE FALSE FALSE FALSE FALSE FALSE FALSE FALSE
[391] FALSE FALSE FALSE FALSE FALSE FALSE FALSE FALSE FALSE TRUE
[401] FALSE FALSE FALSE FALSE FALSE FALSE FALSE FALSE FALSE FALSE
...
[491] FALSE FALSE FALSE FALSE FALSE FALSE FALSE FALSE FALSE
因此,我们可以看到检测到在第一次数据创建时指定的变化点(201,401th)附近的点。
1
可下载资源
关于作者
Kaizong Ye是拓端研究室(TRL)的研究员。在此对他对本文所作的贡献表示诚挚感谢,他在上海财经大学完成了统计学专业的硕士学位,专注人工智能领域。擅长Python.Matlab仿真、视觉处理、神经网络、数据分析。
本文借鉴了作者最近为《R语言数据分析挖掘必知必会 》课堂做的准备。
非常感谢您阅读本文,如需帮助请联系我们!