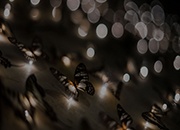
95%置信区间限制非常接近此处的估计线,因为我们已经模拟了具有大样本量的数据集。
有时危险函数不会是恒定的,这将导致累积危险函数的梯度/斜率随时间变化。
可下载资源
危险率函数
让我们模拟R中的一些数据:
n < - 10000
h < - 0.5
t < - -log(runif(n))/ h

该代码模拟了危险函数的存活时间,即常数。
event< - 1 *(t <5)
obstime< - t
obstime [obstime> = 5] < - 5

现在让我们使用R中的生存包绘制估计的生存函数:
survfit < - survfit(Surv(obstime,event)~1)

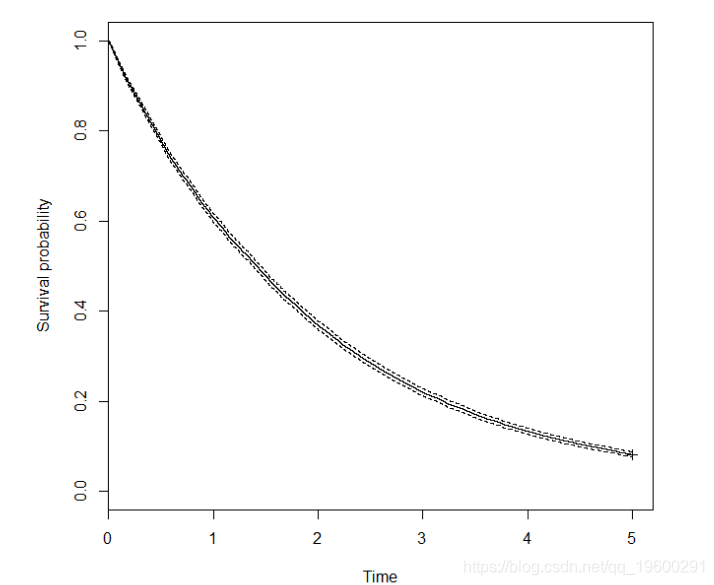

Kaplan-Meier
95%置信区间限制非常接近此处的估计线,因为我们已经模拟了具有大样本量的数据集。
累积危险率函数
为了确定危险函数是否在变化,我们可以绘制累积危险函数,
plot(survfit,fun =“cumhaz”)
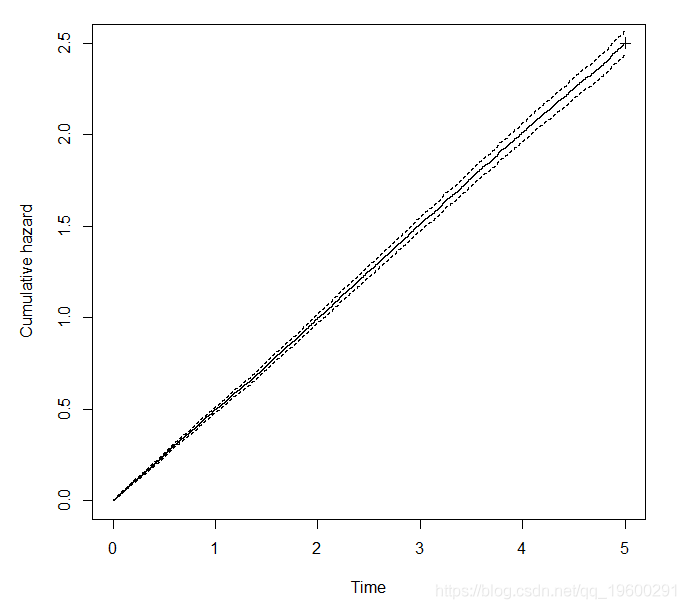

危险变化
有时危险函数不会是恒定的,这将导致累积危险函数的梯度/斜率随时间变化。
我们现在将再次模拟生存时间 :
highrisk < - 1 *(runif(n)<0.5)
h < - 0.5 + highrisk * 1.5
t < - -log(runif(n))/ h
obstime [obstime> = 5] < - 5

我们再次绘制累积危险函数:
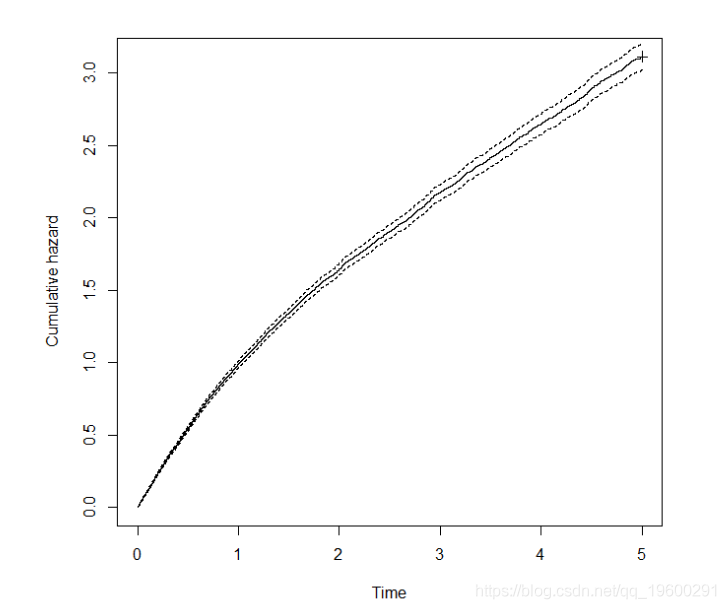

累积危险图,其中样本由50%低风险和50%高风险对象组成
该图的自然解释是受试者经历的危险随着时间的推移而减少,因为累积危险函数的梯度/斜率随时间降低。
改变风险比
在我们比较两组生存率的研究中可能出现同样的问题,例如在比较两种治疗方案的随机试验中。这种比较通常通过估算两组之间的风险比来概括,假设两组的危害比率随着时间的推移是恒定的,使用Cox的比例风险模型。
highrisk < - 1 *(runif(n)<0.5)
治疗< - 1 *(runif(n)<0.5)
t < - -log(runif(n))/ h
事件< - 1 *(t <5)
时间< - t
obstime [obstime> = 5] < - 5

现在让我们分别按治疗组绘制累积危险函数:
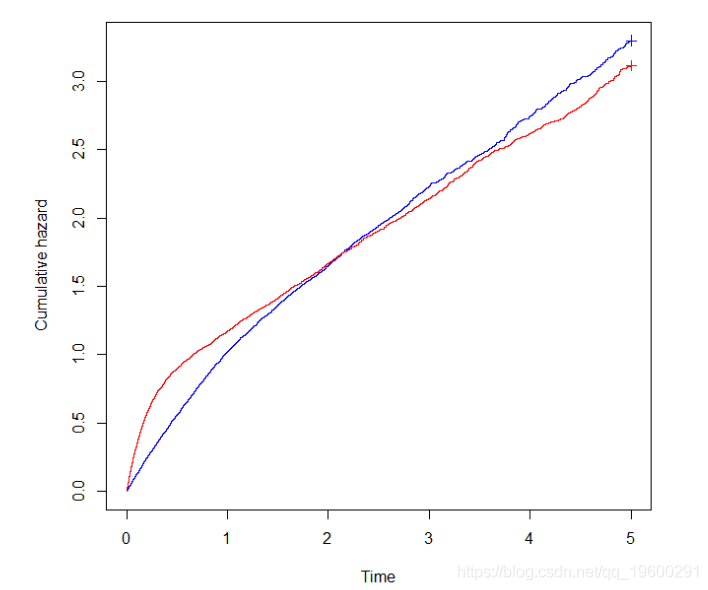