自回归条件异方差(ARCH)模型涉及具有时变异方差的时间序列,其中方差是以特定时间点的现有信息为条件的。
ARCH模型假设时间序列模型中误差项的条件均值是常数(零),与我们迄今为止讨论的非平稳序列不同),但其条件方差不是。
ARCH模型
这样一个模型可以用公式1、2和3来描述。
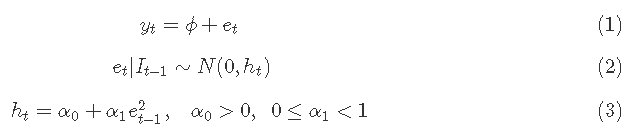
可下载资源
方程4和5给出了测试模型和假设,以测试时间序列中的ARCH效应,其中残差e^t来自于将变量yt回归一个常数,如1,或回归一个常数加上其他回归因子;方程4中的测试可能包括几个滞后项,在这种情况下,无效假设(方程5)是所有这些项都不显著。

无效假设是不存在ARCH效应。检验统计量为
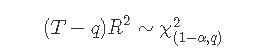
下面的例子使用了数据集,它包含了500个股票收益率的生成观测值。图显示了数据的时间序列图和柱状图。
plot.ts(r)
hist(r)
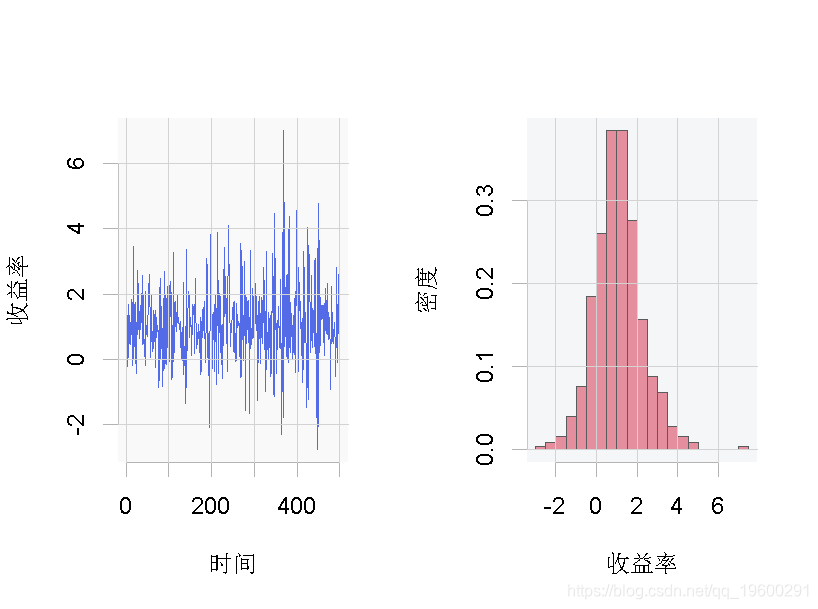
图: 变量 的水平和柱状图
让我们首先对数据集中的变量r一步一步地进行公式4和5中描述的ARCH检验。
summary(yd)
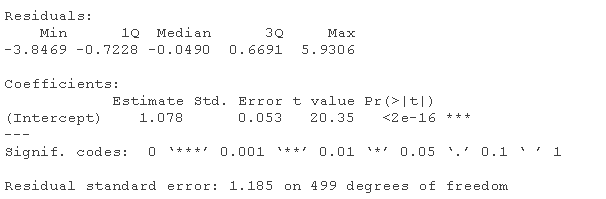
ehsq <- ts(resid(mean)^2)
summary(ARCH)
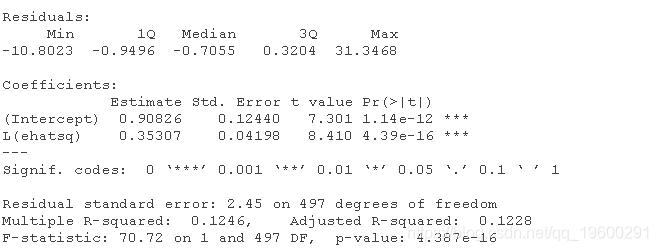
Rsq <- glance(ARCH)\[\[1\]\]
LM <- (T-q)*Rsq
Chicr <- qchisq(1-alpha, q)
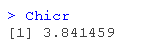
结果是LM统计量,等于62.16,与α=0.05和q=1自由度的临界卡方值进行比较;这个值是χ2(0.95,1)=3.84;这表明拒绝了无效假设,结论是该序列具有ARCH效应。
如果我们不使用一步步的程序,而是使用R的ARCH检验功能之一,也可以得出同样的结论。
ArchTest

函数garch(),当使用order=参数等于c(0,1)时,成为一个ARCH模型。这个函数可以用来估计和绘制方程3中定义的方差ht,如以下代码和图所示。
garch(r,c(0,1))
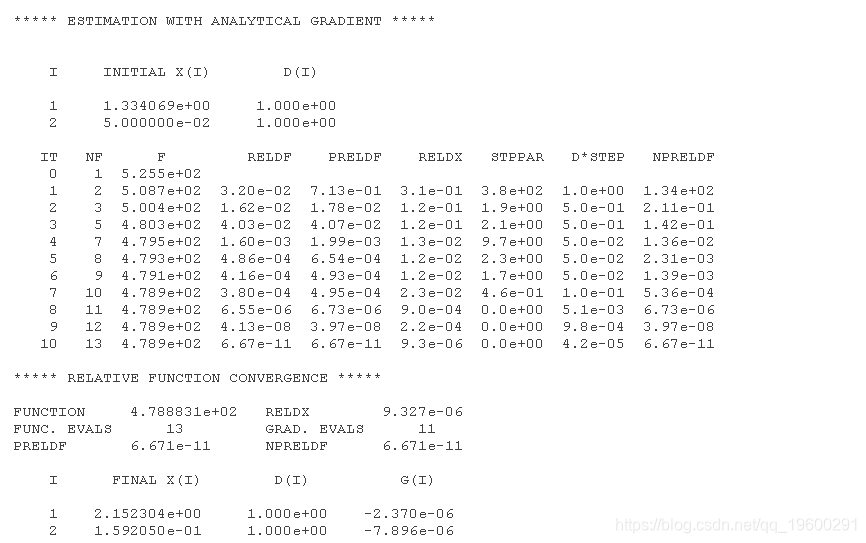
随时关注您喜欢的主题
summary(arch)
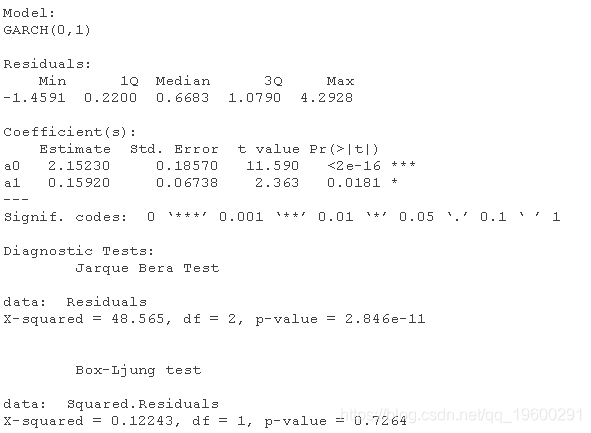
ts(2*fitted.values^2)
plot.ts(hhat)
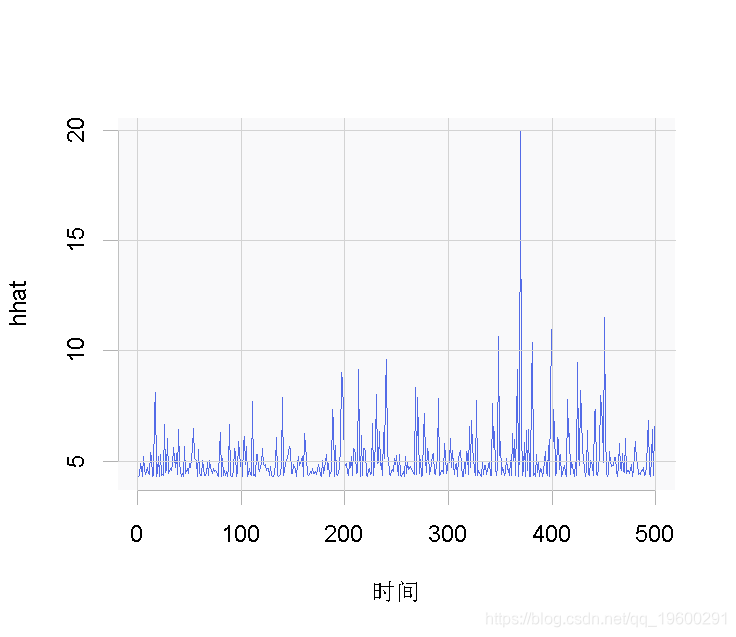
图 对数据集的ARCH(1)方差的估计
GARCH模型
# 使用软件包\`garch\`来建立GARCH模型
fit(spec=garch, data=r)
coef(Fit)

fitted.values
fit$sigma^2)
plot.ts(hhat)
图: 使用数据集的标准GARCH模型(sGARCH)。
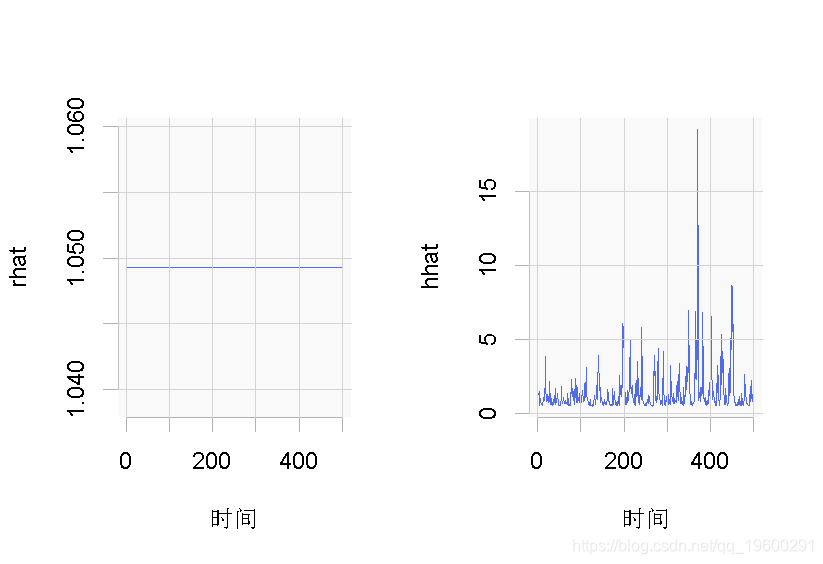
# tGARCH
garchfit(spec, data=r, submodel="TGARCH")
coef(garchfit)

fitted.values
fit$sigma^2)
plot.ts(hhat)
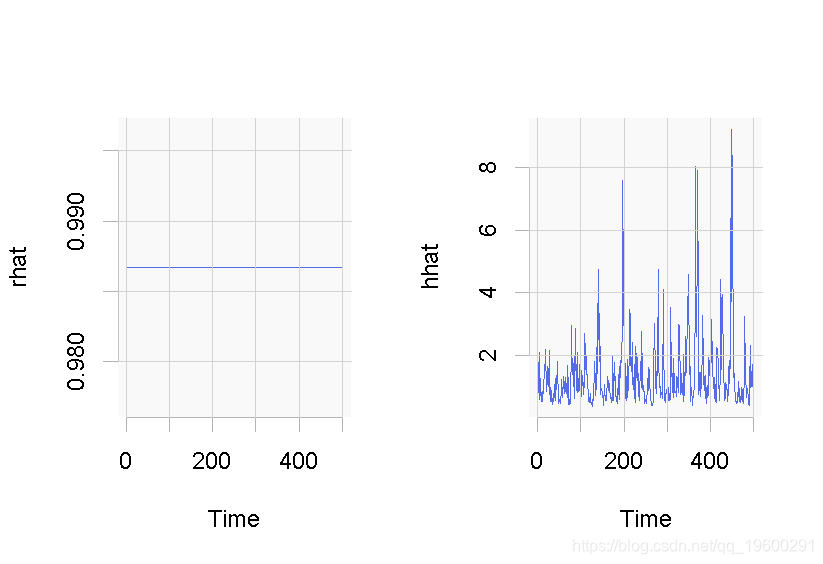
图: 数据集的tGARCH模型
# GARCH-IN-MEAN模型
fit( data=r,
distribution="std",variance=list(model="fGARCH")
coef(garchFit)

fit$fitted.values
fit$sigma^2)
plot.ts(hhat)
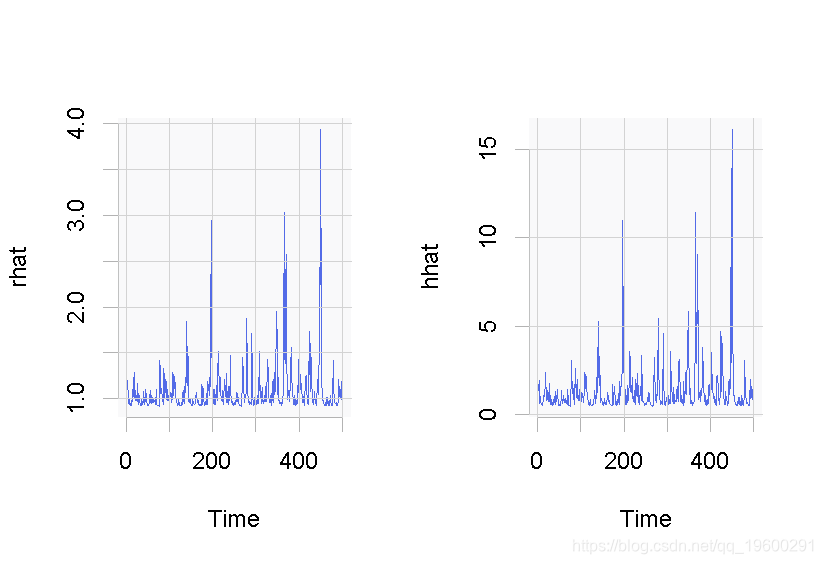
图:使用数据集的GARCH-in-mean模型的一个版本
图显示了GARCH模型的几个版本。预测结果可以通过ugarchboot()来获得。
可下载资源
关于作者
Kaizong Ye是拓端研究室(TRL)的研究员。在此对他对本文所作的贡献表示诚挚感谢,他在上海财经大学完成了统计学专业的硕士学位,专注人工智能领域。擅长Python.Matlab仿真、视觉处理、神经网络、数据分析。
本文借鉴了作者最近为《R语言数据分析挖掘必知必会 》课堂做的准备。
非常感谢您阅读本文,如需帮助请联系我们!