介绍
有限混合模型在应用于数据时非常有用,其中观察来自不同的群体,并且群体隶属关系未知。
模拟数据
首先,我们将模拟一些数据。让我们模拟两个正态分布 – 一个平均值为0,另一个平均值为50,两者的标准差为5。
m1 <- 0
m2 <- 50
sd1 <- sd2 <- 5
N1 <- 100
N2 <- 10
a <- rnorm(n=N1, mean=m1, sd=sd1)
b <- rnorm(n=N2, mean=m2, sd=sd2)

现在让我们将数据“混合”在一起……
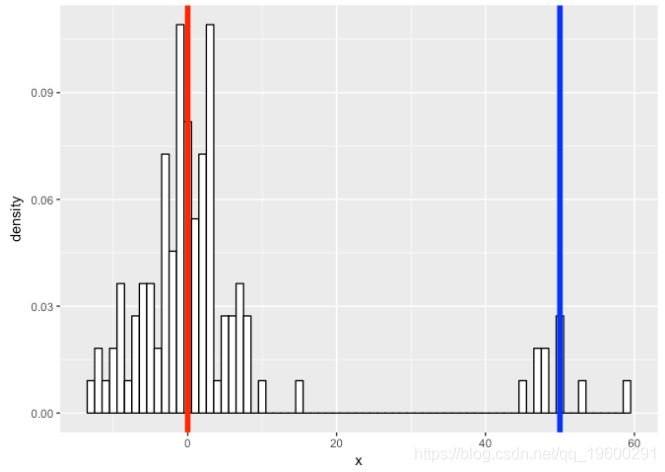

print(table(clusters(flexfit), data$class))
##
## 1 2
## 1 100 0
## 2 0 10

参数怎么样?
cat('pred:', c1[1], '\n')
cat('true:', m1, '\n\n')
cat('pred:', c1[2], '\n')
cat('true:', sd1, '\n\n')
cat('pred:', c2[1], '\n')
cat('true:', m2, '\n\n')
cat('pred:', c2[2], '\n')
cat('true:', sd2, '\n\n')
## pred: -0.5613484
## true: 0
##
## pred: 4.799484
## true: 5
##
## pred: 52.86911
## true: 50
##
## pred: 6.89413
## true: 5

让我们可视化真实数据和我们拟合的混合模型。
ggplot(data) +
geom_histogram(aes(x, ..density..), binwidth = 1, colour = "black", fill = "white") +
stat_function(geom = "line", fun = plot_mix_comps,
args = list(c1[1], c1[2], lam[1]/sum(lam)),
stat_function(geom = "line", fun = plot_mix_comps,
args = list(c2[1], c2[2], lam[2]/sum(lam)),
colour = "blue", lwd = 1.5) +
ylab("Density")

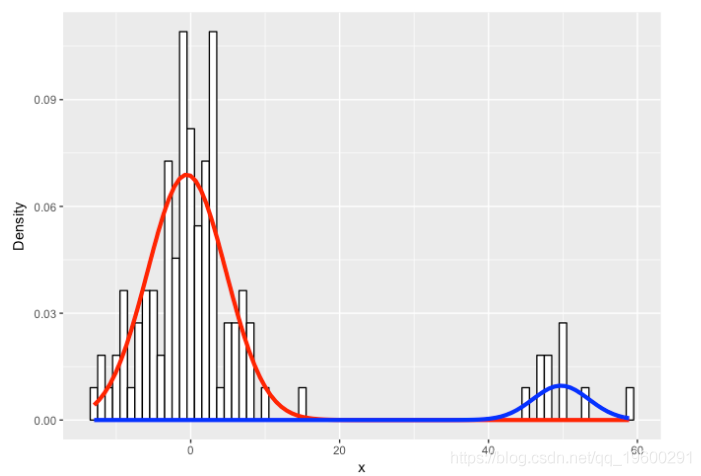

看起来我们做得很好!
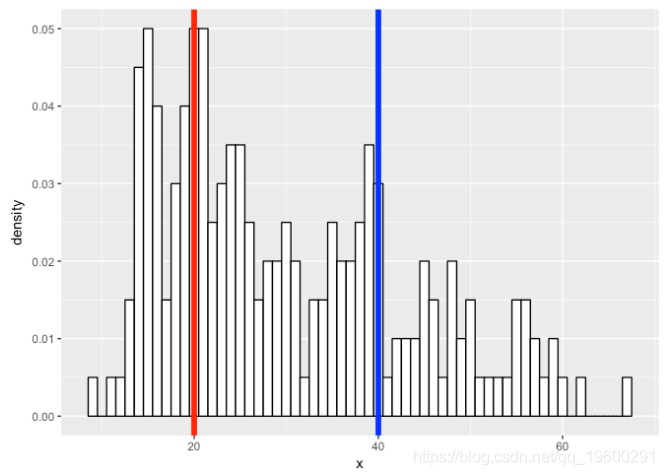

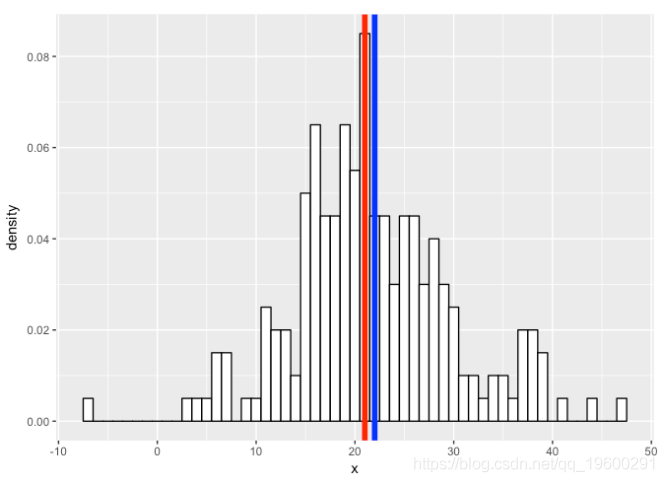

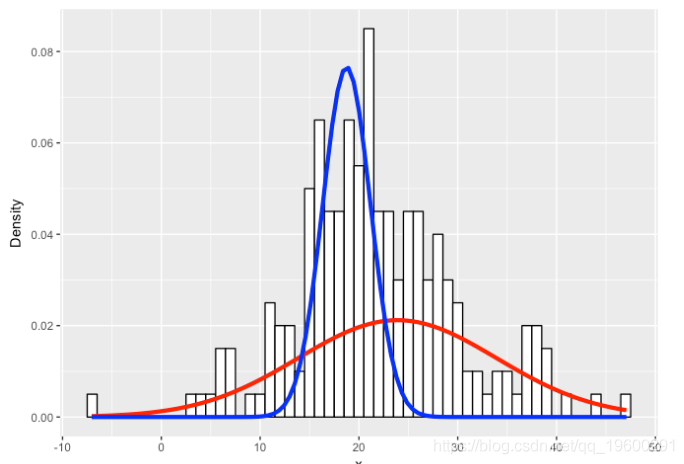

例子
现在,让我们考虑一个花瓣宽度为鸢尾花的真实例子。
p <- ggplot(iris, aes(x = Petal.Width)) +
geom_histogram(aes(x = Petal.Width, ..density..), binwidth = 0.1, colour = "black", fill = "white")
p

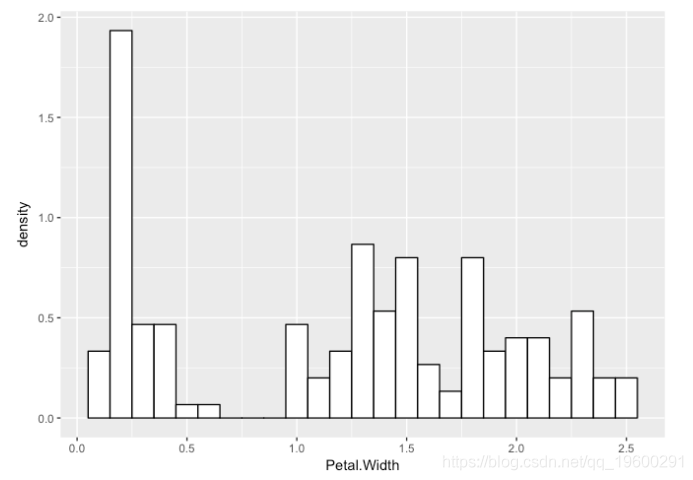

flexfit <- flexmix(Petal.Width ~ 1, data = iris, k = 3, model = list(mo1, mo2, mo3))
print(table(clusters(flexfit), iris$Species))
##
## setosa versicolor virginica
## 1 0 2 46
## 2 0 48 4
## 3 50 0 0
geom_histogram(aes(x = Petal.Width, ..density..), binwidth = 0.1, colour = "black", fill = "white") +
args = list(c1[1], c1[2], lam[1]/sum(lam)),
colour = "red", lwd = 1.5) +
stat_function(geom = "line", fun = plot_mix_comps,
args = list(c2[1], c2[2], lam[2]/sum(lam)),
stat_function(geom = "line", fun = plot_mix_comps,
args = list(c3[1], c3[2], lam[3]/sum(lam)),
colour = "green", lwd = 1.5) +
ylab("Density")

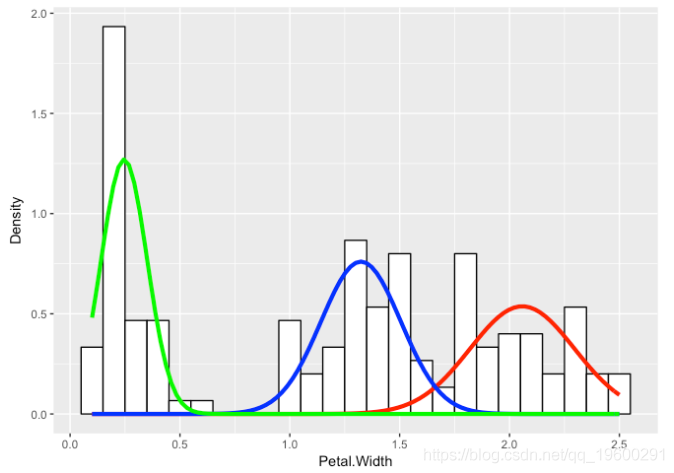

即使我们不知道潜在的物种分配,我们也能够对花瓣宽度的基本分布做出某些陈述 。
非常感谢您阅读本文,有任何问题请在下面留言!
1
1
关于作者
Kaizong Ye是拓端研究室(TRL)的研究员。
本文借鉴了作者最近为《R语言数据分析挖掘必知必会 》课堂做的准备。